AI’s compute expenses are increasing, compute that is driven by incentive networks may be the solution to saving you and your investors millions of dollars
Although decentralized networks exacerbate complexity, they are capable of managing intricate situations. The issue of artificial intelligence’s (AI) insatiable appetite for computing power may be sufficiently intricate to warrant decentralization.
Incentive networks are decentralized networks that foster an “ecosystem” mentality by rewarding individual behavior that benefits the network. The intentionality and mechanisms of an incentive network are distinct from those of a simple ecosystem.
An ecosystem is frequently the result of a fortunate coincidence, wherein a collection of competing forces determines that operating within specific parameters is more advantageous than outside the group. A shared accomplishment is the primary objective of an incentive network from the outset.
However, how does this relate to artificial intelligence? Scalable AI applications are analogous to mechanical devices that generate straightforward responses from extraordinarily vast datasets by employing computational power, similar to the fuel in a vehicle. The more data you transport and the quicker you require the answers, the more gas you consume.
The most intricate and massive AI models consume multiple times as much gas as their simpler counterparts: The compute required to train OpenAI’s GPT-4 was $78 million, while Google’s Gemini Ultra’s cost was $191 million. A system that can dynamically allocate resources and reduce hardware investments to reduce overall costs while remaining impartial to the participants is essential when dealing with such large numbers. This is precisely what incentive networks accomplish.
Related: Blockchain has the potential to mitigate the adverse consequences of AI.
Consequently, incentive networks’ performance is contingent upon the implementation of gamification and token distribution. The incentive network’s creator guarantees that the ecosystem’s value will continue to rise fundamentally and through the actions of its users, rather than relying on the goodwill or stated intentions of others, by encouraging users to engage in behaviors that benefit all participants.
Utilizing a token can refine this incentive system to micro-rewards, thereby establishing a highly sophisticated and intricate economy for the participants to navigate.
There are numerous exceptional examples available. Numeraire (or Numerai) is a hedge fund motivated by data scientists who are compensated for their precise stock market forecasts. Farcaster is a decentralized social network protocol granting users greater content control. This protocol enables users to own their data and maintain a portable social graph that can be transferred across various platforms.
In both instances, they have employed systematic outsourcing to resolve traditional models (trading and publishing) and ancient problems (predicting markets and capturing attention). Both industries in question have experienced substantial consolidation in recent decades and have a relatively low barrier to entry for their participants, at least at the lower end.

It is reasonable to assume that incentive networks would be a beneficial solution to the primary challenge of the 21st century, which is the rapid adoption of AI and its impact on the demand for computing power, also referred to as “compute.”
However, let us return to incentive networks. To be genuinely decentralized, the incentives must promote behavior that is advantageous to the network and be programmed and subsequently accepted by the majority rather than determined and modified by a small number of individuals. They must be equitable yet adaptable, accommodating diversity while being user-centered.
Additionally, they must be straightforward enough to be comprehensible or, at the very least, dependable. This implies that a prodigious quantity of foresight or simple use cases are required. How are such extraordinary vehicles to be created by mere mortals?
Typically, the initial stage in resolving intricate problems is isolating and addressing them individually. This is precisely what layered incentive structures accomplish: they address distinct user responsibilities and contributions separately and incentivize outsized value through outsized rewards.
Dynamic adjustment mechanisms, such as the hyperparameters of a neural network, can also modify the reward based on network conditions to encourage necessary tasks that are underperformed or prevent the unhelpful gaming of the system.
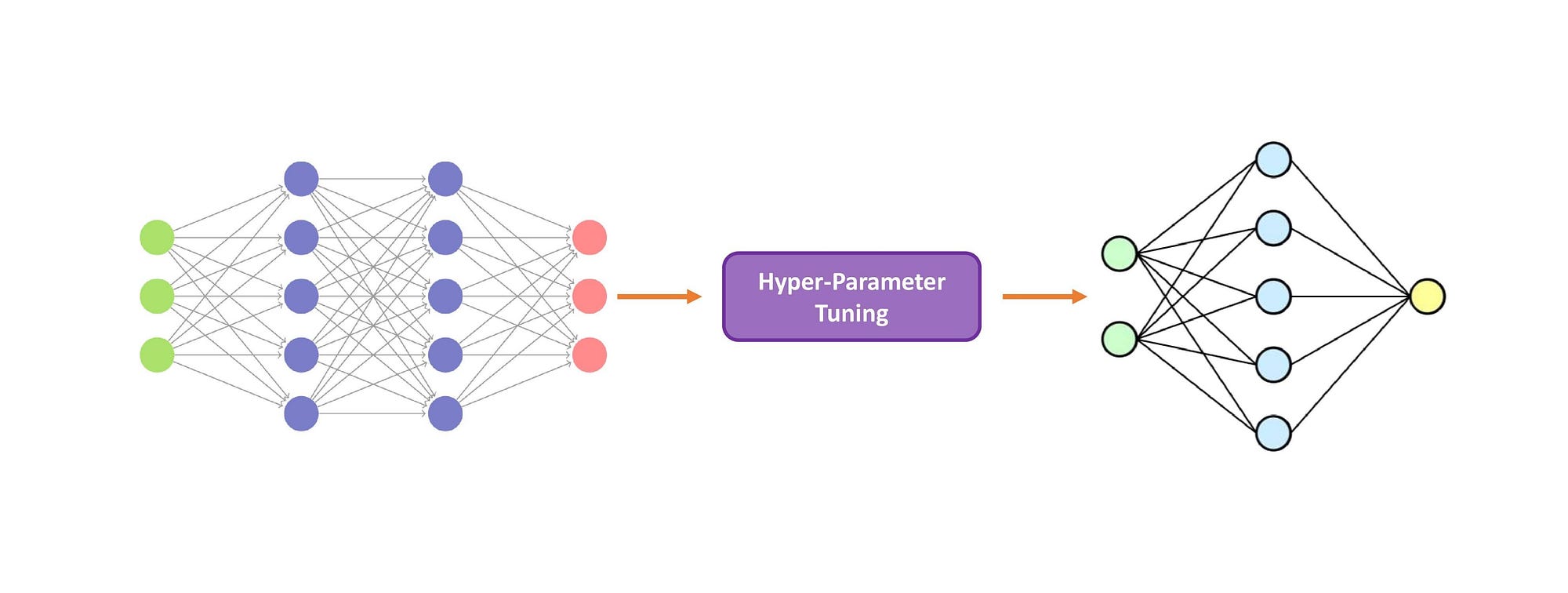
Privacy-focused technology, such as zero-knowledge proofs, can increase participation without fear of reprisal, while reputation systems prioritizing long-term, high-quality, and sustainable actions over quick opportunism bring stability to the user population. Additionally, they can encourage newcomers to put in additional effort to establish themselves.
The objective is to establish an equitable computing system for large and small organizations that adapt to market demands without redundant capacity and can upgrade or repurpose their assets without relying solely on the largest participants. It is a system that facilitates monetizing assets and intellectual property for the benefit of all without favoring any user or provider.
What actions can you take to address this information in a practical manner? DYOR (do your research) remains the same. Please review ventures such as ThoughtAI (THT), Bittensor (TAO), and Ocean Protocol (OCEAN) to determine your level of interest in participating as an investor, builder, or community member.
Alternatively, if you are a more ambitious entrepreneur who aspires to revolutionize an entire industry with AI, delve into the specifics of the training and updating that AI. Incentive-network-driven computing has the potential to save you and your investors millions of dollars. Additionally, it will guarantee that your resources will expand in response to demand if your solution becomes an overwhelming success.
Given that we are discussing the facilitation of AI, it should come as no surprise that the responsibility for administering such a complex system in a decentralized manner should be assigned to an AI.
In the future, we will be able to develop increasingly sophisticated systems that continue to grow and provide value to their users by enabling the incentive network to continuously gather data on the performance and satisfaction of its members, as well as receiving feedback loops from the actual outcomes of the network’s use.
These incentive networks will be able to engage with users individually by utilizing natural language processing, identity systems, and reputation systems. This will enable them to optimize their contribution and rewards based on their unique capabilities, providing them with equipment recommendations or skills training. It is a governance model that prioritizes the human element on a magnitude that has never been achieved.
In the same manner, as with any revolutionary technology, the integration of AI and incentive networks will facilitate increased productivity and the transfer of power from large organizations to individual contributors. It is incumbent upon each of us to remain informed about these advancements and establish an expectation of a world that is superior in theory and more equitable, personal, and effective in practice.