Cyber threats such as identity theft, phishing, data breaches, and financial fraud are becoming increasingly common in Fintech applications
Applications of artificial intelligence (AI) include machine learning, pattern recognition, and natural language processing. These tools have become rescuers that can improve the security and efficiency of procedures. Rapidly scanning huge databases, they can spot anomalies that might point to fraudulent activity.
Let us investigate how AI is essential to improving Fintech applications’ security.
Financial Technology Common Security Risks
- Data Breaches
These organizations keep enormous volumes of personal information, including credit card, bank, and identity numbers. Unauthorized access to this data may result in identity theft, financial fraud, and breaches.
- Phishing Attacks
To get illegal access to financial accounts or personal data, con artists pose as representatives of various organizations.
- Fraudulent Transactions
These are those in which hackers modify systems used for transactions or obtain access to consumer accounts.
- Regulatory Compliance Risks
Fintech companies must operate under laws that safeguard consumer records and prevent money laundering cases since breaking financial regulations can have legal ramifications and cause customers to lose faith.
- Malware and Ransomware
Malware can steal data, compromise systems, and interrupt services. Conversely, ransomware is computer programs that encrypt files and demand payment, jeopardizing the availability and security of financial information.
- Insider Risks
Employees or contractors represent internal risks to fintech companies because they could compromise security, disclose private information, or cause financial harm.
- API vulnerabilities
Since fintech depends on APIs for connection and data sharing, any security holes could be used by criminals to gain access or interfere with services.
Why Can Common Threats Not be Detected by Conventional Security Measures?
Traditionally designed for more stable, less volatile situations, traditional security measures frequently fail in the finance industry. As players use technologies like AI and machine learning, new and developing cyber threats may outsmart these defenses.
In addition, the sophisticated integrations and high transaction rates found in fintech systems call for security solutions that are more efficient at scale and in real-time than many conventional tools.
Besides, the strict regulatory environment in the financial sector is constantly changing. Hence, traditional procedures need to be more adaptable to guarantee ongoing compliance.
AI’s Place in Current Fintech Security
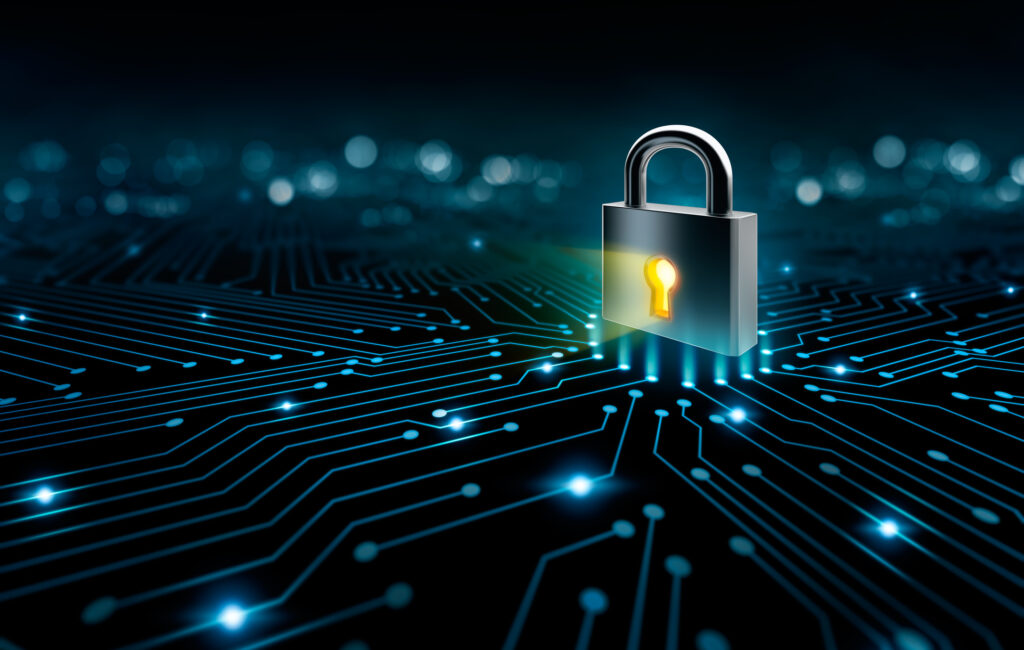
Fraud detection and prevention depend critically on AI’s capacity to spot trends and anomalies. The process by which AI accomplishes this is examined in more depth here, particularly about real-time prevention:
Pattern recognition is a specialty of artificial intelligence systems, especially those trained with machine learning methods. These algorithms are trained to identify fraud by examining previous transaction data, including both valid and fraudulent activity.
During this training, the AI learns to distinguish between typical transaction patterns and those that might indicate fraud.
Anomaly detection is pattern recognition in which the AI seeks out unusual data points. Transactions deviating from a user’s typical spending habits or activities for a specific account could be considered anomalies. AI systems can see these variations and warn fraud analysts of possible fraudulent conduct.
Natural Time Prevention
AI’s ability to function in real-time is among its main benefits in fraud prevention. Traditional fraud detection methods usually use batch processing, which can delay detection and reaction times.
Artificial intelligence modifies this by evaluating transactions as they happen. An AI system can take rapid action upon spotting a possibly fraudulent transaction.
AI systems that are adaptive learn and adjust to new behavioral patterns continually. They may collect fresh data, learn from it, and modify their detection algorithms as needed as fraudsters’ tactics improve. This adaptive learning ability is necessary to keep up with the always-shifting field of fraudulent activity.
AI Resources for Security
Security uses AI technologies since they can learn, adapt, and precisely forecast threats. Several AI security technologies are as follows:
Machine Learning
ML algorithms are very good at analyzing transactional data to identify fraud. They can avoid financial crimes like identity theft and credit card fraud by learning from past fraud trends and spotting minute irregularities that could point to a security breach.
Deep learning is applied in fintech to enhance fraud detection systems that combine voice and facial recognition as biometric authentication techniques to safeguard user accounts and transactions. This technology also makes the analysis of intricate financial data possible, yielding investing insights.
Natural language processing, or NLP, is used in fintech to ensure adherence to regulations like anti-money laundering (AML) requirements and to track and examine consumer interactions for phishing indicators. Chatbots that provide safe client support and help can be built using them.
Predictive analytics examines financial data patterns to help fintech companies anticipate and control risks. This enables proactive asset protection measures by forecasting market trends, credit issues, and even the possibility of criminal activity.
Finding data points, events, or observations that are not typical of a dataset is known as anomaly detection. Unusual data may indicate a severe occurrence, such as a system breakdown or cyberattack.
Automated Incident Response
Fintech platforms can be protected against threats by automated incident response systems that act instantly upon detection of a security event. This covers stopping sales, alerting clients, and implementing security measures to prevent data breaches or financial losses.
What connections do behavior analytics and biometric authentication techniques have with AI?
Continuous multi-factor authentication is supported by a complete security solution created by integrating AI with biometric and behavioral data.
It first verifies IDs, then keeps an eye on user activity, and, in response to anomalies, dynamically looks for more biometric confirmation to guarantee high-level security with the least disruption to the user. In these respects, AI advances:
Biometric Authentication
AI enhances biometric authentication by raising the accuracy and dependability of methods like fingerprint and face recognition.
High-precision deep learning algorithms respond to issues like aging or little fingerprint changes by understanding intricate biometric patterns.
An essential defense against efforts at fraudulent access, they also guarantee authenticity by differentiating between real users and spoof biometrics.
Moreover, biometric data comparison to massive databases in AI-powered solutions enables quick, real-time authentication, expediting the verification process while continuously learning and changing to preserve accuracy over time.
Artificial intelligence (AI) transforms behavior analytics by profiling user interactions to establish baselines for normal behavior, including keystrokes, mouse patterns, and application usage; artificial intelligence (AI) transforms behavior analytics.
It is good at spotting behavioral irregularities and pointing out odd data access as a possible danger. Beyond login, this monitoring enables AI to request re-verification if changes happen during a session.
Moreover, AI assigns user action risk levels, and when high-risk behaviors are detected, security measures are activated. This dynamic method of behavior analytics guarantees a sophisticated, real-time reaction to security problems and vigilant protection throughout user sessions.
Complexity of Fintech Regulatory Requirements
Regulations aimed at defending customers, guaranteeing financial stability, and averting crimes abound in the fintech sector and include GDPR, KYC, and AML guidelines. GDPR mandates stringent data protection and demanding data management procedures for those in the EU and EEA.
Financial institutions must confirm client identities, evaluate risks, and maintain current records to stop illegal activity. Through their requirement that banks disclose anomalous transactions and keep copious financial records, AML laws stop money laundering.
Because these rules are dynamic and adjust to new threats and changes in the industry, fintech companies find compliance challenging. Businesses in many countries must keep up with changing legislation, which requires a lot of money and constant focus to remain out of legal hot water and win over customers.
How Artificial Intelligence Improves Regulatory Compliance
AI is revolutionizing financial compliance by automating data management, ensuring GDPR compliance, and simplifying the KYC process.
Machine learning supports AML due diligence by evaluating data to identify and indicate potential money laundering behavior. AML compliance depends on AI systems’ real-time monitoring and prompt alarms.
Whereas AI-generated reports and NLP analysis of legal texts minimize paperwork, predictive analytics enables proactive adaptation to regulatory changes. Growth is made possible while the consistency and scalability of AI reduce human mistakes.
Compliance officers can concentrate on strategic activities because of automation’s cost and operational savings. AI’s revolutionary influence on regulatory compliance helps fintech companies to comply with regulations swiftly, proactively handle changes, and lower the possibility of fines, thus safeguarding their reputation.
Including AI in Human Supervision
With developments offering to enhance predictive analytics, real-time decision-making, biometric security, regulatory compliance, and quantum computing defenses, the future of AI in fintech security is bright.
However, the best defense plan will combine these cutting-edge AI capabilities with human oversight’s priceless judgment and morality. This well-rounded strategy will guarantee that security is optimized while adhering to more general laws.
Optimizing Strengths and Weaknesses
AI systems are very good at rapidly evaluating data and seeing trends people would miss. But they need to grasp context the same way that people do. Human judgment and AI abilities working together guarantee that judgments are based on context awareness, human experience, and facts.
Ethical Aspects and Bias Reduction
If biases are not carefully monitored, AI systems may unintentionally reinforce them. Establishing ethical guidelines for AI use and monitoring AI systems for biased findings require human oversight. This monitoring guarantees fair and equal fintech security solutions for all demographics.
Adapting to New Threats
As new data is made accessible, AI systems—especially those built on machine learning—need constant training. Upgrading and recalibrating these systems to handle fresh and changing security risks requires human experience.
Final choice Authority
Usually, human specialists should make the final choice for critical judgments with significant ramifications. With this approach, humans control essential security decisions, while AI is a potent instrument for data analysis and suggestion creation.
Finally, some reflections
As a significant gatekeeper in the fintech industry, AI offers sophisticated solutions to counter various cyber threats, including sophisticated financial fraud and data breaches.
AI enhances the security architecture by using machine learning, deep learning, natural language processing, and predictive analytics.
AI combined with biometrics and behavioral analytics ushers in a new era of continuous, multi-factor authentication that preserves user experience while providing strong and sophisticated security.
However, the combination of AI and human supervision guarantees these powerful technologies are employed with the required ethical and contextual awareness.
AI plays a growing role in safeguarding the fintech sector from current and new threats and guaranteeing adherence to the always-evolving regulatory compliance as the industry develops quickly.