As businesses hurry to embrace AI, HPE research indicates that many are falling into an overconfidence trap, disregarding crucial gaps in their plans
Artificial intelligence (AI) has captivated the attention of enterprises across industries, promising dramatic efficiencies, new solutions, and a competitive edge.
However, as businesses rush to adopt this game-changing technology, a sobering reality emerges: many fall into an AI overconfidence trap, missing significant gaps that could jeopardize their AI strategy and investments.
A recent global analysis commissioned by Hewlett Packard Enterprise (HPE) reveals a troubling disparity between organizations’ confidence in their AI readiness and the natural holes in their strategies.

The study, which polled over 2,000 IT leaders from 14 countries, discovered that over half (44%) of respondents felt their companies were adequately prepared to reap the benefits of AI. However, the findings show significant flaws that could jeopardize successful AI transformations.
“There’s no doubt that AI adoption is accelerating, with nearly all IT leaders planning to increase their AI spending over the next year,” said Sylvia Hooks, VP of HPE Aruba Networking.
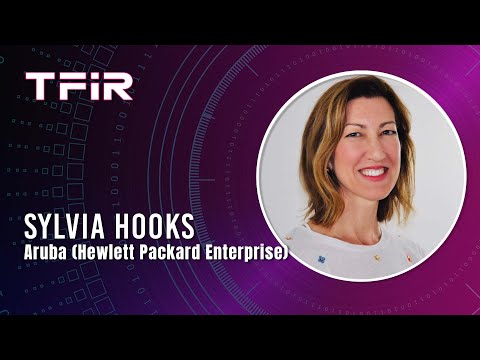
“These findings clearly illustrate the appetite for AI, but they also highlight genuine blind spots that could cause progress to stall if a more comprehensive strategy is not taken. For example, misalignment in strategy and department involvement can prevent organizations from exploiting crucial areas of knowledge, making effective and efficient decisions, and ensuring a holistic AI roadmap serves all sectors of the business equally.”
Data: The Fuel for AI Success
Strong AI performance depends on the quality of the data inputs, which businesses recognize by naming data management as one of the most crucial components for AI success.
However, the analysis reveals unacceptably low data maturity levels. Only 7% of organizations can perform real-time data pushes and pulls, enabling innovation and monetising external data. Only 26% have implemented data governance models and can perform advanced analytics.
Less than six out of ten respondents claimed their organization is fully capable of handling crucial phases of data preparation for AI models, such as accessing (59%), storing (57%), processing (55%), and recovering (51%).
This difference risks slowing down the AI model generation process and increases the likelihood of incorrect insights and a negative return on investment (ROI).
A significant knowledge gap has emerged while confidence levels appear to be high in network infrastructure and compute capacity to accommodate AI traffic and workloads.
Less than half of IT leaders confessed to thoroughly understanding the requirements for various AI workloads such as training, tuning, and inference.
This lack of understanding raises significant concerns regarding organizations’ capacity to accurately allocate resources, potentially resulting in performance bottlenecks, inefficiencies, and suboptimal outcomes.
The paper identifies fragmentation in businesses’ AI initiatives. Over a quarter (28%) of IT leaders viewed their organization’s overall AI approach as “fragmented,” with 35% developing separate AI strategies for individual areas and 32% setting disparate targets.
This walled approach impedes cross-functional collaboration and synergy and increases the danger of duplicating efforts and misaligned priorities.
Much more concerning is the apparent disregard for ethical and compliance standards. IT leaders rated legal/compliance (13%) and ethics (11%) as the least important for AI success. Surprisingly, nearly one in every four organizations (22%) does not include legal teams in their AI strategy discussions.
Risks of Overconfidence
The repercussions of ignoring these blind spots are far-reaching and potentially harmful. Businesses that need a firm AI ethics policy risk building models that do not meet proper compliance and diversity requirements, leading to negative brand reputation, sales losses, hefty fines, and legal disputes.
Furthermore, the quality of AI model outcomes is directly proportional to the quality of the data they use. With data maturity levels staying low and 50% of IT leaders admitting to a lack of complete understanding of IT infrastructure demands throughout the AI lifecycle, the risk of producing ineffective models, including the effects of AI hallucinations, rises considerably.
Furthermore, the massive power demands of AI models might contribute to unwarranted increases in data centre carbon emissions, reducing the ROI on capital investments in AI and harming a company’s brand.
As companies traverse the world of AI, taking a comprehensive and thoughtful strategy is critical. AI can transform data into meaningful insights across every device on the network, from on-premises model training and tweaking in colocation or the public cloud to edge inference.
Businesses must, however, carefully weigh the ambition to be an early adopter with the risk of failing to appreciate the gaps across the AI lifecycle fully. Failure to do so can result in massive capital investments with a poor ROI, undercutting AI’s very benefits.
Recognizing and fixing these blind spots is essential for AI development. Organizations must prioritize data maturity and integrate data management methods with AI goals.
They must also thoroughly understand computing and networking requirements throughout the AI lifecycle, ensuring proper resource provisioning and infrastructure scalability.
“AI is the most data and power-intensive workload of our time, and to effectively deliver on the promise of Gen AI, solutions must be hybrid by design and built with a modern AI architecture,” said
Dr Eng Lim Goh, senior vice president of data and Artificial Intelligence at HPE
“From on-premises model training and tweaking to edge inference, Gen AI can turn data into insights from every device on the network. However, organizations must carefully balance the benefits of being a first mover against the risk of not understanding the gaps across the AI lifecycle; otherwise, substantial capital investments can result in a negative ROI.”