A new AI tool, Tyche, helps diagnose diseases by accounting for ambiguity in medical scans, providing multiple possible interpretations for doctors.
Segmentation in biomedicine entails the annotation of pixels that correspond to significant structures within a medical image, such as cells or organs. Clinicians can receive assistance from artificial intelligence models emphasizing pixels that may indicate a particular disease or anomaly.
Nevertheless, these models generally offer a singular solution, whereas the challenge of segmenting medical images is frequently complex and multifaceted. In the case of a lung CT image, five proficient human annotators who may have differing opinions regarding the presence or magnitude of the nodule’s borders could produce five distinct segmentations.
“Oppositions can be beneficial when making decisions.” “Even the awareness that a medical image contains an element of uncertainty can affect a person’s decision-making process; therefore, it is crucial to account for this uncertainty,” advises Marianne Rakic, a doctoral candidate in computer science at MIT.
Rakic co-authors a paper with colleagues from Massachusetts General Hospital, MIT, the Broad Institute of MIT, and Harvard, which presents a novel artificial intelligence (AI) instrument capable of discerning the ambiguity inherent in a medical image.
The system called Tyche, named after the Greek deity associated with chance, generates numerous plausible segmentations that emphasize marginally distinct regions of a medical image. A user can determine the number of options that Tyche displays and choose the one that best suits their needs.
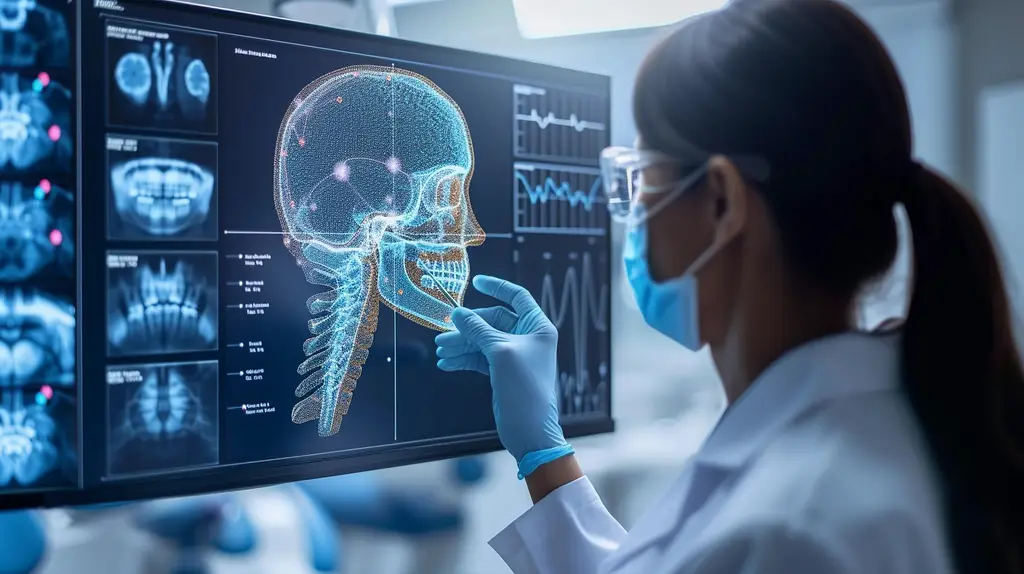
Tyche can, and this is crucial, perform novel segmentation tasks without requiring retraining. Training is an exhaustive process that necessitates the presentation of numerous examples to a model and demands a significant amount of machine-learning expertise.
As it does not require retraining, clinicians and biomedical researchers may find Tyche more straightforward than alternative approaches. It can be used “out of the box” for an extensive range of applications, including detecting abnormalities in a brain MRI or identifying lesions in a lung X-ray.
In essence, this system has the potential to enhance diagnostic capabilities and contribute to biomedical research by highlighting potentially vital data that alternative AI tools may overlook.
Ambiguity needs more scholarly investigation. “You should probably pay attention if your model completely misses a nodule that three experts say is there and two experts say is not,” advises senior author Adrian Dalca, a research scientist at the MIT Computer Science and Artificial Intelligence Laboratory (CSAIL) and an assistant professor at Harvard Medical School and MGH.
Beth Cimini, associate director for bioimage analysis at the Broad Institute; Jose Javier Gonzalez Ortiz, PhD ’23; Hallee Wong, a graduate student in electrical engineering and computer science; John Guttag, the Dugald C. Jackson Professor of Computer Science and Electrical Engineering, are among their co-authors. Tyche has been designated as a highlight presentation at the IEEE Conference on Computer Vision and Pattern Recognition, where Rakic will deliver the presentation.
Confronting ambiguity
AI systems commonly employ neural networks for medical image segmentation. Neural networks process data and are loosely inspired by the human brain. They are machine-learning models composed of numerous interconnected layers of nodes or neurons.
Following discussions with Broad Institute and MGH collaborators who utilize these systems, the researchers identified two significant limitations restricting their efficacy. For even a marginally different segmentation task, the models must be retrained due to their inability to account for uncertainty.
Some approaches attempt to circumvent a single pitfall, but Rakic states that addressing both issues with a single solution has proved particularly challenging.
“To account for ambiguity, one must frequently employ an extraordinarily complex model.” “Our objective with the proposed method is to facilitate rapid prediction using a relatively small model while maintaining ease of use,” she explains.
Tyche was constructed by researchers modifying a basic neural network architecture.
Before anything else, a user provides Tyche with a few examples illustrating the segmentation task. Multiple images of cardiac lesions extracted from an MRI could serve as examples; these images would have been segmented by distinct human experts, allowing the model to acquire proficiency in the task and identify instances of ambiguity.
The researchers discovered that a “context set” consisting of sixteen example images is sufficient for the model to generate accurate predictions; however, the number of examples employed is not restricted. Due to the context set, Tyche can resolve novel tasks without requiring retraining.
The researchers modified the neural network to generate multiple predictions from a single medical image input and the context set to account for Tyche’s uncertainty. They modified the network’s layers so that the candidate segmentations generated at each stage could “communicate” with the examples in the context set as the data moved from layer to layer.
Thus, the model can guarantee that each prospective segmentation is unique while accomplishing the objective.
“It is similar to tossing dice. “Either one may reappear if your model is capable of rolling a two, three, or four but is unaware that you already possess a two and a four,” she explains.
Additionally, they altered the training procedure to incentivize the model and optimize the quality of its most accurate prediction.
In response to a user’s request for five predictions, Tyche will generate all five medical image segmentations, each of which may be superior to the others.
Additionally, the researchers created a Tyche variant compatible with a pre-trained model currently in use to segment medical images. Tyche enables the model to generate multiple candidates by performing minor image transformations.
Better and more rapid forecasts
Upon subjecting Tyche to datasets containing annotated medical images, the researchers discovered that its predictions adequately reflected the variety of human annotators. Its most accurate predictions surpassed those of the baseline models. Additionally, Tyche outperformed the majority of models.
Rakic states, “Generating multiple candidates and ensuring they are distinct gives you a significant advantage.”
According to the researchers, Tyche was also capable of surpassing the performance of more intricate models trained on voluminous, specialized datasets.
They intend to experiment with a more versatile context set, which could consist of text or various forms of images. Furthermore, they are interested in investigating techniques that could enhance Tyche’s worst predictions and improve the system’s ability to suggest the most qualified candidates for segmentation.
The National Institutes of Health, the Eric and Wendy Schmidt Center at the Broad Institute of MIT and Harvard, and Quanta Computer have partially funded this research.